Data annotation technology, while an essential component of artificial intelligence (AI) and machine learning (ML), often raises questions about its legitimacy, significance, and applications. As AI becomes increasingly integrated into industries such as healthcare, finance, retail, and autonomous systems, data annotation plays a foundational role in enabling machines to understand and process data accurately. This article explores whether data annotation technology is legitimate by examining its importance, widespread adoption, accuracy, and real-world applications.
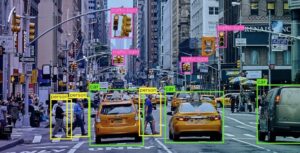
What Is Data Annotation Technology?
Data annotation involves labeling data—such as images, text, or audio—to make it usable for machine learning models. AI systems rely on annotated data to recognize patterns, learn from them, and make informed predictions. For example:
- In image recognition, annotated datasets help AI systems identify objects, faces, or scenes.
- In natural language processing (NLP), labeled text is used to teach AI to understand sentiment, context, or intent.
- In autonomous driving, annotated videos assist AI in identifying pedestrians, road signs, and other vehicles.
Data annotation technology includes tools and platforms that facilitate this labeling process. These tools often incorporate automation, enabling faster, more accurate annotations by leveraging algorithms and pre-trained models.
The Importance of Data Annotation
1. Foundation of Machine Learning
Machine learning models are only as good as the data they are trained on. Annotated datasets provide the ground truth that helps models learn to classify, predict, or analyze new information. Without accurate annotations, AI systems would struggle to make reliable decisions.
2. Improving AI Accuracy
Annotation ensures that the data used for training is labeled consistently and correctly. This accuracy is crucial in high-stakes applications such as medical diagnosis, fraud detection, and autonomous vehicles, where even small errors can have significant consequences.
3. Enabling Real-World Applications
Data annotation bridges the gap between raw data and actionable AI applications. Whether it’s powering voice assistants, improving product recommendations, or enabling precision medicine, annotated data is the backbone of these technologies.
Is Data Annotation Tech Legit mate?
To address the legitimacy of Is Data Annotation Tech Legit, it’s essential to consider several factors:
1. Widespread Adoption by Major Industries
Major tech companies like Google, Amazon, and Microsoft rely heavily on data annotation to power their AI products. The widespread use of annotation platforms and tools in diverse industries is a testament to their legitimacy. From healthcare AI systems analyzing X-rays to retail algorithms personalizing customer experiences, data annotation is an integral part of modern technology.
2. Accuracy and Quality Control
Reputable data annotation platforms invest heavily in quality control measures. These include multi-layered validation processes, AI-assisted labeling, and human-in-the-loop systems that ensure annotations are precise. As a result, high-quality annotations drive the accuracy of AI models, reinforcing the legitimacy of the technology.
3. Transparency and Ethics
Legitimate data annotation technology providers prioritize transparency regarding their processes and ethics. They comply with data privacy regulations such as GDPR and CCPA, ensuring that the data used for annotation is obtained ethically and processed securely. This transparency builds trust among businesses and users.
4. Scalability and Customization
The flexibility of annotation platforms to scale and customize their services for various industries also highlights their credibility. From small startups to multinational corporations, businesses can leverage these tools to meet specific project needs, further demonstrating the reliability of data annotation technology.
Challenges and Concerns
Despite its legitimacy, data annotation technology does face some challenges:
1. Dependence on Human Annotators
Many annotation tasks still require human expertise, especially in nuanced areas such as medical imaging or sentiment analysis. Ensuring consistency and avoiding human bias are ongoing challenges.
2. High Costs and Time Consumption
Manually annotating large datasets can be time-consuming and expensive. While automation tools alleviate some of this burden, achieving the necessary level of accuracy often requires significant investment.
3. Ethical Considerations
The use of sensitive data for annotation, such as personal medical records or surveillance footage, raises ethical concerns. Ensuring that these processes align with privacy laws and ethical standards is critical for maintaining trust.
Applications of Data Annotation Technology
The practical applications of data annotation technology underscore its value and legitimacy:
1. Healthcare
In healthcare, annotated data is revolutionizing diagnostics and treatment planning. AI systems trained on annotated medical images can detect diseases such as cancer, while NLP models analyze patient records for insights into treatment outcomes.
2. Autonomous Vehicles
Self-driving cars rely on annotated datasets to understand their environment. This includes identifying pedestrians, traffic lights, road signs, and other vehicles. Is Data Annotation Tech Legit ensures the accuracy and safety of these systems.
3. E-Commerce
Retailers use annotated data to enhance customer experiences. From personalized product recommendations to advanced search capabilities, annotated data drives the algorithms that power these features.
4. Natural Language Processing
In NLP, annotated text data is used for tasks like language translation, sentiment analysis, and chatbots. Companies such as OpenAI and Google heavily rely on annotated data to improve the conversational abilities of AI systems.
5. Security and Surveillance
Annotated video and image data are used in facial recognition, anomaly detection, and threat identification systems, making security measures more robust.
Future of Data Annotation Technology
The future of data annotation technology looks promising, driven by advancements in automation and AI. Key trends include:
1. Increased Automation
Automation tools that use AI to assist in labeling are becoming more sophisticated. This reduces the reliance on human annotators while maintaining accuracy.
2. Focus on Ethical AI
As the demand for annotated data grows, there is an increasing focus on ensuring ethical practices. This includes transparent data usage policies, fair compensation for annotators, and strict adherence to privacy laws.
3. Emergence of Niche Applications
As AI penetrates new sectors, the need for domain-specific annotated data will grow. From agriculture to space exploration, annotation technology will adapt to meet these emerging needs.
Conclusion
Data annotation technology is undoubtedly legitimate, serving as the cornerstone of AI and machine learning. Its widespread adoption, emphasis on accuracy, and ability to power real-world applications underscore its importance. While challenges such as ethical concerns and dependency on human annotators persist, advancements in automation and a focus on ethical AI practices continue to bolster its credibility.
As industries increasingly rely on AI to solve complex problems, the role of data annotation will only grow. Far from being a niche or questionable practice, data annotation technology is a vital, legitimate force shaping the future of artificial intelligence.